In the heart of the Fourth Industrial Revolution, manufacturing is undergoing a profound transformation, driven by the power of artificial intelligence (AI). At the forefront of this revolution stands machine learning, a subset of AI that enables machines to learn from data and improve their performance over time. From predictive maintenance to quality control, machine learning is revolutionizing every aspect of the manufacturing process, offering unmatched efficiency, quality, and cost savings.
Consider this: a factory floor where machines can predict their own failures before they occur, preventing costly downtime and ensuring uninterrupted production. Or imagine a quality control system that can identify defects in products with near-perfect accuracy, reducing waste and improving customer satisfaction. These are just a few examples of how machine learning is reshaping the manufacturing industry. In this blog, we will explore the world of machine learning and its applications in manufacturing. We’ll discuss the benefits, challenges, and future trends of this groundbreaking technology, so you can understand the impact of machine learning for manufacturers and gain a competitive edge and position yourself for success.
What is Machine Learning?
Machine learning is a subset of artificial intelligence that enables machines to learn from data and improve their performance over time. Unlike traditional programming, where rules are explicitly defined, machine learning algorithms can identify patterns and make predictions without being explicitly programmed.
There are several types of machine learning:
- Supervised learning: Involves training a model on labeled data, where the input and desired output are provided. For example, in manufacturing, a model can be trained to predict whether a product will be defective based on sensor data collected during the production process.
- Unsupervised learning: Is about training a model on unlabeled data, where the model must discover patterns and relationships within the data. For example, a manufacturing plant can use unsupervised learning to identify groups of similar products based on their characteristics.
- Reinforcement learning: consists of training a model to make decisions based on rewards and punishments. The model learns by trial and error, maximizing its rewards over time. For example, a robot arm can learn to assemble products more efficiently by receiving rewards for successful assembly and punishments for errors.
Machine learning algorithms can be used to solve a wide range of problems in manufacturing, from predicting equipment failures to optimizing production processes.
Benefits of Machine Learning in Manufacturing
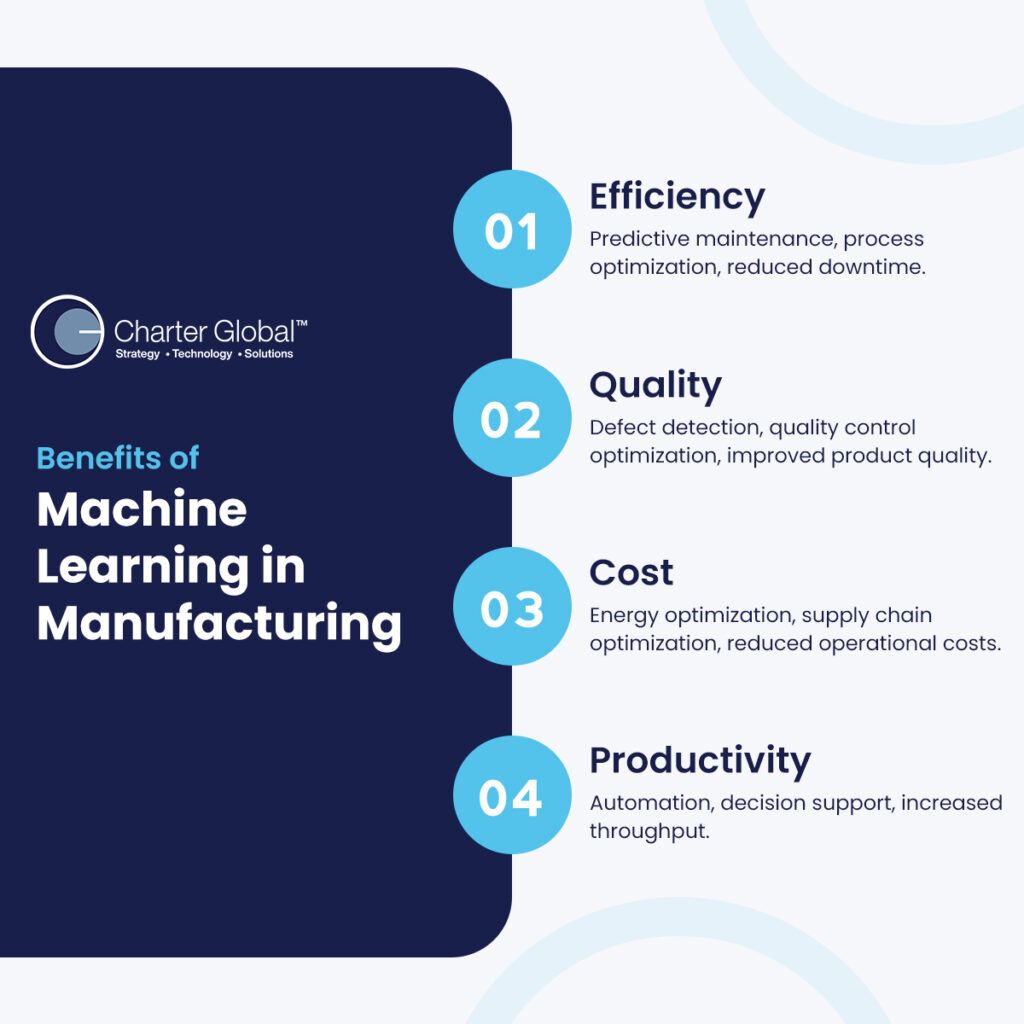
Machine learning offers numerous benefits to manufacturers, including:
Improved Efficiency
- Predictive Maintenance: ML algorithms can analyze historical data from sensors and equipment to predict potential failures before they occur. This allows for proactive maintenance, reducing downtime and ensuring uninterrupted production.
- Process Optimization: ML can be used to optimize manufacturing processes, such as scheduling, inventory management, and energy consumption. For example, ML algorithms can analyze production data to identify bottlenecks and optimize workflows, leading to increased efficiency and reduced costs.
Enhanced Quality
- Defect Detection: ML-powered computer vision systems can inspect products for defects with greater accuracy than human inspectors, reducing the risk of defective products reaching the market.
- Quality Control Optimization: ML can be used to optimize quality control processes, such as identifying root causes of defects and implementing corrective actions. This can help to improve product quality and reduce waste.
Reduced Costs
- Energy Optimization: Machine Learning can be used to optimize energy consumption in manufacturing facilities, reducing energy costs and environmental impact. For example, ML algorithms can analyze energy usage data to identify areas for improvement and implement energy-saving measures.
- Supply Chain Optimization: ML can help optimize supply chains by improving demand forecasting, inventory management, and transportation planning. This can reduce costs associated with excess inventory, stockouts, and inefficient transportation.
Increased Productivity
- Automation: ML automates repetitive tasks, freeing up workers to focus on more complex and value-added activities. For example, robots equipped with ML algorithms can perform tasks such as assembly, welding, and packaging with greater speed and accuracy than humans.
- Decision Support: Machine Learning can also provide decision support to manufacturers by analyzing data and providing insights into complex problems. For example, ML algorithms can analyze customer data to identify trends and preferences, enabling manufacturers to tailor their products and services to meet customer needs.
ML pinpoints defect causes from vast data. It automatically filters signals, reducing manual investigation by 99% and cutting root cause analysis time from weeks to hours. – acerta.ai
Specific Applications of Machine Learning in Manufacturing
Machine learning is being applied to a wide range of manufacturing processes, such as;
Predictive Maintenance
- Predicting Equipment Failures: ML algorithms can analyze sensor data from equipment to predict potential failures before they occur, allowing for preventive maintenance and reducing downtime.
- Optimizing Maintenance Schedules: ML can be used to optimize maintenance schedules based on equipment usage, condition, and historical failure data. This can help to reduce maintenance costs and improve equipment reliability.
Quality Control
- Defect Detection: Machine learning-enabled vision systems can identify product flaws more effectively, minimizing the risk of defective items being shipped.
- Root Cause Analysis: ML can be used to identify the root causes of quality problems, enabling manufacturers to implement corrective actions and prevent future defects.
Supply Chain Optimization
- Demand Forecasting: ML algorithms can analyze historical sales data to predict future demand for products, enabling manufacturers to optimize inventory levels and production planning.
- Transportation Optimization: ML can be used to optimize transportation routes and schedules, reducing transportation costs and improving delivery times.
Product Design and Development
- Simulation and Optimization: ML can be used to simulate product performance and optimize designs for factors such as cost, quality, and sustainability.
These are just a few examples of how machine learning is being applied to manufacturing. As the technology continues to evolve, we can expect to see even more innovative applications in the years to come.
Manufacturers can use machine learning to predict and prevent production problems. By analyzing assembly data, ML models can identify key points contributing to failures and recommend optimal part pairings to minimize rework. This approach has reduced rework rates for axle assemblies by 65%. -acerta.ai
Challenges and Considerations
While machine learning definitely offers significant benefits to manufacturing, there are challenges and considerations too to be addressed:
- Data Quality and Quantity: High-quality and sufficient data is essential for training effective ML models. Collecting and cleaning data can be a time-consuming and resource-intensive process.
- Privacy and Security: The use of ML in manufacturing involves collecting and processing large amounts of data, which raises concerns about data privacy and security. Manufacturers must implement robust data protection measures to safeguard sensitive information.
- Implementation Costs: Implementing ML solutions can involve significant upfront costs, including hardware, software, and skilled personnel. Manufacturers must carefully evaluate the potential benefits and costs of ML before making investment decisions.
- Skill Gap: There is a growing demand for skilled professionals with expertise in machine learning and data science. Manufacturers may face challenges in finding and retaining talent with the necessary skills.
To overcome these challenges, manufacturers should invest in data quality initiatives, implement strong data security measures, carefully evaluate the costs and benefits of ML, and invest in training and development to build a skilled workforce.
Now more than 80% of companies and businesses need employees with machine learning skills. It’s essential for fewer equipment breakdowns, smoother production flow, and ultimately, a significant increase in output. -masterofcode.com
Future Trends and Outlook
The future of machine learning in manufacturing is bright, with several emerging trends and technologies shaping the industry:
- Artificial Intelligence (AI): AI is rapidly evolving and will continue to play a significant role in manufacturing. AI-powered systems can automate more complex tasks, improve decision-making, and enhance overall efficiency.
- Internet of Things (IoT): IoT devices can generate vast amounts of data that can be used to train ML models and optimize manufacturing processes. The integration of IoT and ML will enable manufacturers to gain deeper insights into their operations and make more informed decisions.
- Edge Computing: Edge computing involves processing data closer to the source, reducing latency and improving real-time decision-making. This technology will be crucial for implementing ML applications in manufacturing environments where low latency is essential.
- Explainable AI: As ML models become more complex, there is a growing need for explainable AI, which allows users to understand how models arrive at their decisions. This will be important for building trust in ML systems and ensuring accountability.
The convergence of these technologies will drive the adoption of machine learning in manufacturing, leading to even greater benefits and innovations in the years to come.
Conclusion
Machine learning is revolutionizing the manufacturing industry, offering significant benefits in terms of efficiency, quality, cost, and productivity. And by leveraging the power of ML, manufacturers can stay competitive in today’s rapidly changing market and position themselves for success in the future.
If you’re a manufacturer looking to improve your operations, consider partnering with Charter Global. Our team of experts can help you identify and implement the right ML solutions to meet your specific needs. With our expertise in manufacturing and technology, we can help you leverage the full potential of machine learning and drive your business forward.
Contact Charter Global for a Consultation.
Or mail us at [email protected] or call +1 770.326.9933